Anneke Damen
Systematic reviews and meta analyses of prediction model studies: methods and applications
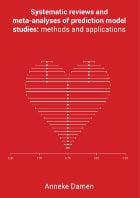
- Datum
- (Co) promotoren
- 14-06-2018
- prof.dr. K.G.M. Moons, prof.dr. R.J.P.M. Scholten, dr. T.P.A. Debray, dr. L. Hooft
Samenvatting
Prediction models are becoming increasingly important in clinical practice. Unfortunately, research on prediction models is often not reproducible and the usefulness of most models in clinical practice is unclear. This is because researchers do not always use the recommended methods for developing or validating a prediction model. Furthermore, often numerous models exist for the same target population or condition. Systematic reviews have therefore become important to appraise and summarize the current evidence on existing prediction models in a specific clinical field. Although ample guidance exists for systematic reviews of interventions and diagnostic tests, guidance for systematic reviews and meta-analyses of prediction models is lacking. In this thesis we present guidance for systematic reviews of prediction models and meta-analysis of their predictive performance. This guidance is subsequently applied in systematic reviews and meta-analyses in the field of cardiovascular disease (CVD). We showed that there is an overabundance of prediction models for cardiovascular disease. Most of these models predicted the risk of coronary heart disease (CHD) or CVD over 10 years and the majority of models consisted of a similar set of core predictors, including age, gender, smoking, diabetes, blood pressure, and blood cholesterol. Substantial heterogeneity in predictor and outcome definitions was observed between models, and important clinical and methodological information, necessary to externally validate the model or even apply it in clinical practice, were often missing. Only one third of the available models was externally validated, and therefore the usefulness in clinical practice of most models remains unclear. We further focused on three often advocated prognostic models (Framingham Wilson, Framingham ATP III, and Pooled Cohort Equations (PCE)) for the prediction of 10-year risk of CHD or CVD and summarized their predictive performance in terms of discrimination and calibration. There was considerable heterogeneity in the predictive performance between studies, likely due to differences in eligibility criteria, and population characteristics. On average, however, all models discriminate comparable well and all models overestimate the 10-year risk of CHD and CVD. One way to improve the predictive performance of available prediction models, is to add new predictors to the model. We showed that adding extra biomarkers to a prognostic model with traditional predictors including age, smoking, and blood pressure, did result in very limited improvement of performance of this model for predicting 10-year risk of CHD. Another problem in the field of prediction model studies, is poor reporting. We showed that more than half of the items that are considered essential for transparent reporting of a prediction model in the TRIPOD statement were not or inadequately reported. In the last part, we studied sources of heterogeneity in the predictive performance of prognostic models from various clinical fields. Using a meta-epidemiological approach, we found that this heterogeneity is mainly associated with variation in population aspects and noticed some indications for an association with predictor and outcome measurement methods.