Constanza Andaur Navarro
Quality of machine learning prediction models in healthcare
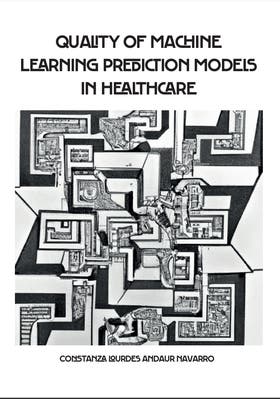
- Datum
- (Co) promotoren
- 16-05-2023
- Promotors: Prof. dr. K.G.M. Moons, Prof. dr. L. Hooft
Copromotor: Dr. J.A.A.G. Damen
Samenvatting
For the implementation of valuable prediction models in clinical practice, properly conducted and well reported studies on early stages of model development and validation are essential. We systematically reviewed the adherence of 152 studies on machine learning-based prediction models to the 22-item checklist with the minimum standards for high quality reporting, TRIPOD. Overall, articles adhered to a median of 38.7% of applicable TRIPOD items. We identified that TRIPOD requires new items to cover AI-related aspects. We systematically reviewed the included studies for 15 spin practices and 11 poor reporting standards. A considerable number of studies lack a pre-specified protocol, make claims of clinical applicability (without further validation), and limitations are neither reported nor discussed in the context of previously developed models. Given that a first approach to spin evaluation using a classification scheme for studies on prognostic factors proved to be inefficient, we present SPIN-PM, a new framework for spin identification tailored to studies on prediction models. We provide a detailed overview of the study design, modelling strategies, and performance measures reported in studies on machine learning-based prediction models. Most studies reported only the development of prediction models and focused on binary outcomes. Within the 152 studies, we evaluated 522 models (on average 9.4 models per study), in which the most common modelling algorithms used were support vector machine and random forest. We comprehensively reviewed the methodological quality and risk of bias of studies on prediction models developed using machine learning techniques. We applied PROBAST to 152 studies on model development and 19 external validations. Of these 171 analyses, 148 were rated at high risk of bias. Efforts to improve the design, conduct, reporting, and validation of such studies are necessary to boost the introduction of machine learning based prediction models into clinical practice. We compared the absolute risk probabilities of three different modelling techniques: logistic regression, random forest, and support vector machine. For the last two techniques, we applied two different implementation methods within the package ‘caret’ in the statistical software R. Using logistic regression as benchmark, we showed that risk probabilities for deep venous thrombosis vary substantially between modelling techniques and implementation methods. TRIPOD and PROBAST were published to facilitate the critical appraisal of studies on diagnostic and prognostic prediction models. We described the five stages for the development of both extensions to machine learning-based models. The systematic reviews presented in this thesis compromised stage one. A survey using the Delphi methodology constitute stage 2 and the results are briefly discussed in Chapter 12. In conclusion, we have thoroughly evaluated the methodological conduct and reporting of studies on machine learning-based prediction models. The findings will contribute to the development of both PROBAST-AI and TRIPOD-AI. Furthermore, we have proposed a framework for spin identification in studies on prediction models, SPIN-PM. Overall, we upgraded the current guidance for quality evaluation and interpretation of findings in studies on prediction models, potentially helping reduce vague and biased research outputs.