Jeroen Hoogland
Prognostic and treatment effect modeling in medical research
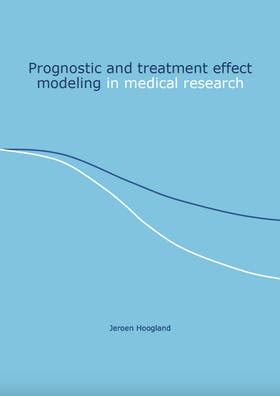
- Datum
- (Co) promotoren
- 11-07-2023
- prof. dr. K.G.M. Moons
prof. dr. M.M. Rovers
dr. T.P.A. Debray
dr. J. in 't Hout
Samenvatting
Prediction models are increasingly used in the medical domain, benefitting from the increased availability of digital healthcare data and accessible software implementations. Two important applications of such models are (i) predicting the likely course of a medical condition (also known as prognosis) and (ii) predicting of the consequences of a particular treatment decision. In both cases, the goal is often to go beyond the prediction of population averages towards more personalized predictions. This dissertation provides a biostatistical perspective on such prediction models for prognosis and treatment effects. Specific methodological contributions include methods to handle missing data points during the validation of prediction models and their application in practice. New developments are also described regarding methods that help strike the right balance between model complexity and the size of the available data. Furthermore, the differences between associative and causal prediction modeling are explored in depth, and guidance is provided for the development of prediction models for predicting personalized treatment effects based on randomized trial data. models for treatment effect heterogeneity based on randomized trial data is provided. Subsequently, the evaluation of the quality of such models is described, including a novel approach to measure prediction performance. Finally, an applied chapter describes the modeling of both prognosis and treatment effect in a context where individual patient level data are available from multiple studies.