Romin Pajouheshnia
Prognostic Research in Treated Populations
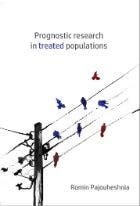
- Datum
- (Co) promotoren
- 30-10-2018
- prof.dr. K.G.M. Moons, prof.dr. R.H.H. Groenwold, dr. L.M. Peelen
Samenvatting
For both diagnostic and prognostic prediction models to effectively support clinical practice, they must provide accurate, clinically relevant and interpretable predictions. This thesis examines potential sources of variation in the predictive performance of prognostic prediction models when applied in new individuals. Attention is primarily given to the issue of treatment use in studies that develop or externally validate prognostic models, how this can affect the accuracy and generalizability of such prediction models, and possible methodological solutions. In chapter 2, the performance of three cardiovascular disease (CVD) prognostic models: Framingham Wilson, the ATP III model and the ACC/AHA Pooled Cohort Equations was systematically reviewed. 112 external validations were included and scored for risk of bias. Meta-analyses found the c-statistic greatly varied across the studies and the three models generally overestimated CVD risk. Meta-regression analysis indicated that greater variation in patient characteristics and their clinical measurements may be associated with a larger c-statistic. In chapter 3, a typology for treatments was proposed to better understand when and how they should be taken into account when developing or validating a prognostic model. A systematic review, including 302 articles, found that nearly one-third of studies reporting prognostic models for CVD did not report any information on treatment use, and treatments after baseline were not reported. In chapter 4, the mechanisms underlying the effect of treatment use on the results of a validation study were examined in detail. Following this, four different approaches to account for unwanted treatment effects on measures of model performance were examined theoretically, and compared in a simulation study. An inverse probability weighting (IPW) approach was found to perform well when treatment was non-random. Chapter 5 compares approaches to account for the effects of time-varying treatment use when developing a prognostic model. A case study investigated more sophisticated methods using real patient data. Seven approaches to develop a prognostic model were compared. While the use of different approaches did alter the coefficients in the prognostic model and the risk predictions made by the model, this did not translate to a noticeable difference in model performance. Chapter 6 addresses differences in the measurement of predictors across settings. A general expression relating error in the measurement of a continuous predictor to the area under the ROC curve (AUC) of a prediction model was defined, and applied in a clinical case study. Differences in predictor measurement were found to impact on model performance across settings. Chapter 7 provides recommendations for “best practices” when developing or validating prognostic models using data from randomized clinical trials (RCTs). Benefits of RCT data were identified: data completeness, data quality, randomized treatments and detailed meta-data. Limitations were explored: selective population, non-representative predictor measurements, extraneous trial effects, short-term and surrogate outcomes, sample size and trial participant consent for re-use of the data. Finally, the recommendations made throughout this thesis are integrated within a framework for defining and linking the aims, estimands and estimators of a prediction study, in order to improve the design and interpretation of future prediction model studies.